When it comes to dissertations and capstones, one crucial aspect that often perplexes students is the data analysis process. Dissertation data analysis serves as the backbone of your research, providing insights that will guide your conclusions and contribute to the body of knowledge in your field. However, mastering this endeavor requires more than just the ability to crunch numbers; it demands a strategic and intentional approach that unravels the secrets concealed within your data.
Embracing data analysis within your dissertation entails exploring, interpreting, and making sense of the information you have gathered. This meticulous process allows you to identify patterns, establish relationships, and draw meaningful conclusions from your research. Consequently, it is essential to approach dissertation data analysis with the mindset of a detective, tirelessly seeking clues and unraveling the mysteries that lie beneath the surface of your data.
To navigate this often complex journey, it is crucial to have a comprehensive understanding of the different tools and methods available for data analysis. From statistical software packages to qualitative analysis techniques, there are various ways to make sense of your data. By familiarizing yourself with these tools and methods, you can select the most appropriate ones for your research questions, ensuring that your data analysis process is both rigorous and reliable.
In the following sections of this article, we will delve into the key steps and considerations involved in mastering dissertation data analysis. We will explore the importance of data preparation, the various analysis techniques at your disposal, and the effective communication of your findings. By demystifying this critical aspect of your research journey, we aim to equip you with the necessary skills and knowledge to confidently navigate the intricacies of dissertation data analysis. So, let us embark on this enlightening journey together, unraveling the secrets and mastering the art of dissertation data analysis.
Importance of Dissertation Data Analysis
Dissertation data analysis is a crucial component of both dissertations and capstones. It plays a significant role in unraveling the secrets hidden within the data and shedding light on the research questions or hypotheses posed by the researchers. Through meticulous analysis, researchers can extract meaningful insights, draw valid conclusions, and contribute new knowledge to their respective fields.
Dissertation Proposal Help
Data analysis serves as the backbone of any research study, as it allows researchers to examine and interpret their collected data in a systematic and organized manner. It provides a structured framework for understanding the relationships, patterns, and trends that may exist within the data. By employing appropriate statistical tests and analytical techniques, researchers can explore complex relationships, measure variables, and validate their findings.
One of the primary reasons why data analysis is of paramount importance is that it helps researchers make informed decisions based on empirical evidence. From identifying significant associations to detecting statistical differences, the analysis ensures that the research outcomes are rooted in objective data rather than subjective interpretations. This not only adds credibility to the research but also enables other scholars to build upon the findings, thus fostering the growth and advancement of knowledge in the field.
Furthermore, dissertation data analysis enables researchers to address their research questions or hypotheses effectively. By examining the data through different lenses and perspectives, researchers can validate or refute their initial assumptions, thereby contributing to the ongoing scholarly discourse. The analysis also aids in identifying any limitations or gaps in the research, allowing researchers to propose recommendations for future studies.
In conclusion, dissertation data analysis is an integral part of any research project. It helps researchers unlock the secrets hidden within the data and provides a solid foundation for drawing meaningful conclusions. By unraveling the complexities of the data, researchers can make evidence-based decisions, expand the boundaries of knowledge, and contribute to their respective fields of study.
Key Steps for Successful Data Analysis
In order to master dissertation data analysis and unravel its secrets, it is important to follow a systematic approach. By breaking down the process into key steps, you can ensure a successful data analysis for your dissertations and capstones. Here are three crucial steps to consider:
Data Collection and Preparation
Before diving into the analysis, it is essential to collect relevant data and prepare it for examination. This involves identifying the sources of data and organizing it in a structured manner. Clean and accurate data is vital for meaningful analysis, so make sure to remove any errors, inconsistencies, or duplicates. By having a well-prepared dataset, you set the foundation for a successful analysis.Choosing the Right Analysis Techniques
Once your data is ready, the next step is to determine the most suitable analysis techniques. Consider the research questions and objectives of your dissertation, as they will guide your selection. There are various methods available, such as statistical tests, regression analysis, thematic analysis, or content analysis. By carefully choosing the appropriate techniques, you can gather meaningful insights from your data.Interpreting and Presenting Results
After conducting the analysis, it is crucial to interpret and present the results effectively. Take the time to analyze the findings in relation to your research questions and objectives. Identify patterns, trends, and relationships within the data. Use visualizations, such as charts or graphs, to present your results in a clear and concise manner. This not only helps you understand the implications of your analysis but also enables others to comprehend and evaluate your work.
By following these key steps, you can navigate the complex task of dissertation data analysis more effectively. Remember to approach the process systematically, ensuring data quality, choosing appropriate analysis techniques, and presenting your findings in a coherent manner. With these insights, you will be well on your way to mastering the art of data analysis for dissertations and capstones.
Common Challenges in Dissertation Data Analysis
Data analysis is a crucial component of any dissertation or capstone project. However, researchers often encounter various challenges when undertaking this process. In this section, we will explore some of the common difficulties faced during dissertation data analysis.
Limited Sample Size: One of the key challenges researchers face is having a small sample size for analysis. This limitation can affect the generalizability of the findings and can make it challenging to draw robust conclusions. It is important for researchers to acknowledge this limitation and consider its impact when interpreting the results.
Data Quality Issues: Another common challenge is dealing with data quality issues. Researchers may encounter missing data, outliers, or inaccuracies in their dataset, which can affect the validity and reliability of the analysis. Addressing data quality problems requires careful data cleaning, imputation, and validation techniques to ensure the accuracy of the results.
Complex Statistical Techniques: Dissertation data analysis often involves using complex statistical techniques to analyze the data and test research hypotheses. Researchers without a strong statistical background may find it challenging to select and implement the appropriate statistical tests. Seeking assistance from a statistician or utilizing specialized software can help overcome this challenge and ensure the accurate analysis of the data.
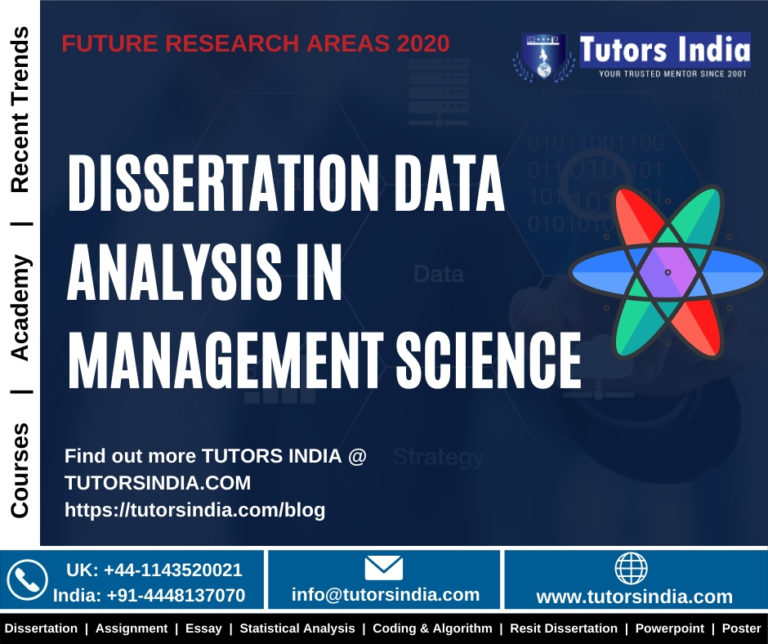
In conclusion, dissertation data analysis presents several challenges that researchers must navigate. These challenges include limited sample sizes, data quality issues, and the need for sophisticated statistical techniques. Overcoming these hurdles requires careful consideration and application of appropriate methodologies to ensure reliable and valid research findings.